Clinical Trials Transformed by Today's Technology Will Empower Tomorrow's Medical Breakthroughs
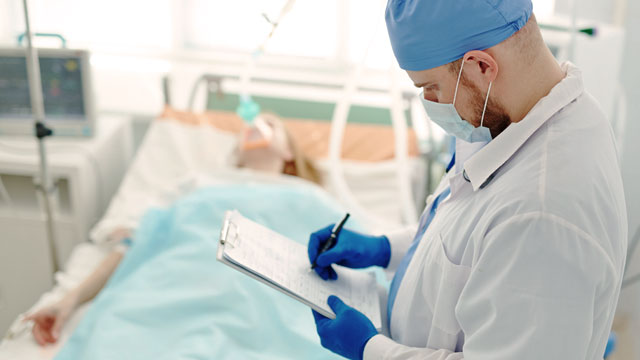
Formally phased and rigorously conducted clinical trials are the gold standard by which medicines and devices are evaluated for safety and efficacy.
As the pace of discovery accelerates, so has the number of clinical studies – from 2,100 in 2000 to nearly 500,000 in April 2024.
Yet traditional clinical trials face significant challenges ranging from recruitment delays and extended timelines to a lack of representative patients and spiraling costs. Trials can take upwards of seven years – with delays causing up to $8 million daily in lost revenue.
The good news: Powered by AI-enabled technologies, real-world data (RWD) and real-world evidence (RWE) remove time, cost and variability from clinical research – facilitating speed to value, a better patient experience and improved outcomes across populations.
Recruitment: More Efficiently Identifying Participants
About 80 percent of trials fail to meet enrollment targets and timelines, making recruitment the most common culprit of delays.
Precise inclusion/exclusion criteria and the advent of personalized medicines mean that trials require specific participants to prove the effectiveness of therapies. However, the complexity of personalization creates additional hurdles in meeting enrollment and eligibility requirements. Complicating matters, prospective participants’ medical records may be in non-digitized, narrative form, scattered across providers with little to no connectivity, making the right candidates difficult to spot in electronic data pulls that must wade through a morass of patient data. This can tip the risk-return equation – often such that trial sponsors divert resources elsewhere.
Data and technology innovations support recruitment with rapid, accurate, at-scale screening. In November, the National Institutes of Health (NIH) released results of a study on TrialGPT, a new AI algorithm developed by researchers at the NIH National Library of Medicine and the National Cancer Institute. TrialGPT processes patients’ medical and demographic information and pairs them with trials for which they are eligible. In the pilot study, the tool expedited the matchmaking process by more than 40 percent.
Elsewhere, natural language processing (NLP) is already lauded as a transformative AI technology for clinical trials innovation. NLP can mine millions of medical records for unstructured data that is not visible in a “checked box” in the electronic medical record. For instance, a trial therapy may be limited to cancer patients in stages 3 or 4 of the disease, yet the structured record may only indicate a cancer diagnosis, whereas the disease stage is communicated in the physician notes. Using NLP, research organizations can use AI to mine both sources of intelligence and more rapidly find the right candidates for the therapy as opposed to combing through physician notes by hand. For clinical trials, that’s a massive time saver that can accelerate the process, boost accuracy and help monitor intervention safety and efficacy.
Sponsors leveraging these capabilities have improved recruitment by 1,800 percent and tripled enrollment over baseline projections – significantly reducing enrollment periods and study costs.
Securing Representation Across Demographics and Conditions
Broad patient participation in U.S. clinical trials is a public health imperative. Women remain underrepresented as do patients in rural areas, various racial/ethnic groups and patients with certain medical conditions. Access, enrollment practices and medical mistrust drive this troubling lack of diversity.
Underrepresentation hurts care quality and outcomes for affected populations. For instance, research shows less than 5 percent of adult cancer patients enroll in cancer clinical trials, and that lack of participation diversity can lead to uneven outcomes in cancer care. Ultimately, a medical breakthrough left unstudied in a population may become a breakthrough denied based on social determinants, geographic location or other disparities.
In 2022, Congress required that sponsors submit trial diversity action plans (DAPs) to the U.S. Food and Drug Administration (FDA). Such plans “… must lay out trial enrollment goals organized by race, ethnicity, sex and age group,” and “… should consider how the medical product’s intended use population is distributed according to these demographics.”
RWD/RWE and innovative technologies allow broad patient identification and enrollment, engagement with a wider care network, and patient discovery in complete therapeutic areas. AI-driven NLP:
- Accelerates medical records analyses across traditionally unreached communities;
- Pinpoints enrollment obstacles;
- Reveals strategies to reach/engage populations;
- Identifies the right patients for the right trials; and
- Helps these patients apply and participate.
Best-in-class datasets mobilize RWD for evidence-based and population-based analyses of drugs, devices, disease states and more. Patient-level data, from age to Z codes help illustrate a patient’s comprehensive health profile, can be tokenized and linked with other specialized sources. This enables actionable insights into demographics and disease prevalence across populations, informing enrollment goals and rationales.
Trial Design: Delivering a Better Patient Experience at Lower Cost
For decades, site selection has favored experience and principal investigator (PI) relationships over key metrics such as volume, eligibility, speed and proximity to targeted enrollees. Site-driven design intensifies the significant challenges of patient recruitment.
Because the site-driven model funnels limited candidates to one geographic location, it increases costs (i.e., travel expenses) and reduces data applicability, as participants are often not representative of the targeted population. Sponsors must hope enough eligible candidates are reasonably close to the location, and inequities are frequent – especially in areas that lack the right targeted participants for complete results.
In this respect, a site-driven approach generalizes data due to unequal representation of certain groups, including rural residents, women and patients with comorbidities.
Decentralized clinical trials (DCTs) help address the problem, delivering accessibility, reduced costs and a better patient experience. Designed to augment or replace the traditional model, DCTs use RWD/RWE and other technologies to apply proven historical performance to a location-agnostic context, bringing the trial to patients via telehealth, remote monitoring and/or local settings. Leveraging predictive analytics of sites’ past performance, DCTs recruit based on where patients live instead of where PI relationships exist.
Synthetic control arms (SCAs) can also streamline studies and ease patient burden. From a scientific standpoint, placebo-controlled trials are widely accepted as the optimal approach. However, this view does not consider the soaring cost of trials nor the ethical considerations of giving sick patients an inactive placebo. Consider a gene transfer trial for late infantile neuronal ceroid lipofuscinosis (today known as CLN2 disease), a fatal condition in children. In this scenario, a traditional control group would require:
- Performing a sham surgery on sick children
- Dividing a small number of patients into experimental and placebo groups
- Raising the financial stakes of an already high-stakes study
- Lengthening the runway to statistically relevant data
The CLN2 study may be an extreme example, yet many sponsors already recognize the power of SCAs to supplement or replace traditional control arms.
SCAs use de-identified patient data (i.e., external control data) from pre-existing datasets, mimicking patients who would otherwise be recruited – then compare the data to an actual group receiving a treatment to evaluate effectiveness.
SCAs can reduce the number of patients needed by up to 50 percent, decreasing trial duration and cost. SCAs can also improve the patient experience and outcomes by enabling all study participants to receive the treatment.
The FDA’s Role: Advancing Clinical Trials
Regulatory agencies are increasingly integrating RWD/RWE, allowing for flexible and faster review.
This trend drove the FDA’s August 2023 and December 2023 guidance on the use of RWD/RWE for regulatory decisions on drugs/biologics (kickstarted by the 21st Century Cures Act). Combined with trial research, RWE can shorten the process and fuel FDA approvals.
The FDA’s commitment to keeping regulatory pace with technological advancements is commendable. We recommend that the agency also:
- Issue clear guidance on patient-consent and data-sharing requirements in trials, particularly considering promising applications for RWD in DCTs and SCAs;
- Capture, monitor and manage pre-specification activities in drug development; and
- Affirm that Pre-Approval Information Exchange (PIE) is applicable in the case of DCTs.
Final Thoughts
The potential to transform clinical trials is within our grasp.
For the research community, long-overdue changes can modernize the sector for greater effectiveness, equity and efficiency – and improved outcomes. Patients counting on access to innovations don’t have time to waste.
RWD/RWE powered by AI in the service of passion for discovery holds a bright future for us all.